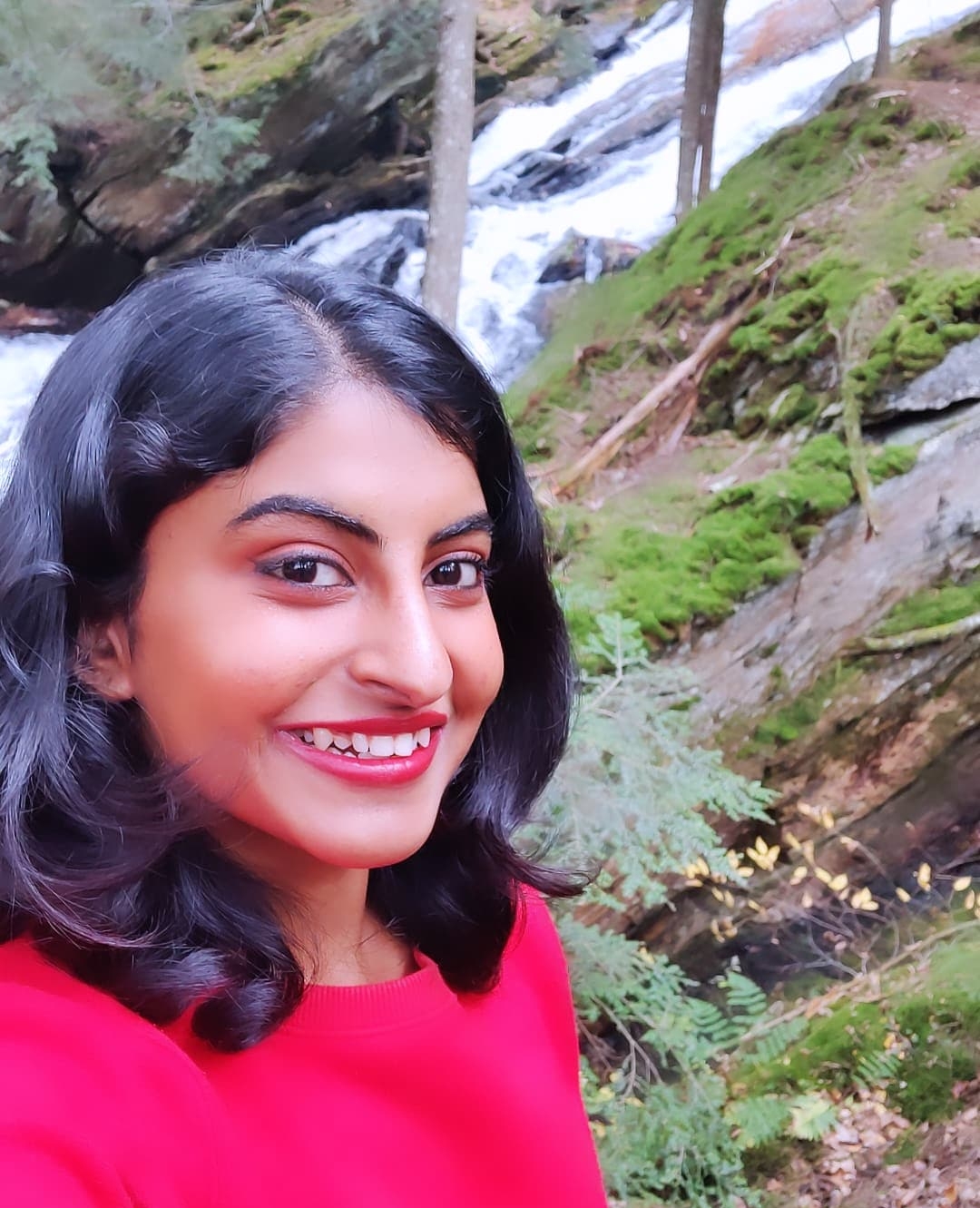
CS PhD Student
GenAI | LLMs | Diffusion Models
- Blacksburg, VA
- Virginia Tech
- Website
- Google Scholar
- Github
- X (formerly Twitter)
Publications
For an up-to-date list of my research papers, please see my Google Scholar.
2024
Context Canvas: Enhancing Text-to-Image Diffusion Models with Knowledge Graph-Based RAG
Kavana Venkatesh, Yusuf Dalva , Ismini Lourentzou , Pinar Yanardag
In arXiv preprint arXiv:2412.09614
We introduce a novel approach to enhance the capabilities of text-to-image models by incorporating a graph-based RAG. Our system dynamically retrieves detailed character information and relational data from the knowledge graph, enabling the generation of visually accurate and contextually rich images. This capability significantly improves upon the limitations of existing T2I models, which often struggle with the accurate depiction of complex or culturally specific subjects due to dataset constraints. Furthermore, we propose a novel self-correcting mechanism for text-to-image models to ensure consistency and fidelity in visual outputs, leveraging the rich context from the graph to guide corrections. Our qualitative and quantitative experiments demonstrate that Context Canvas significantly enhances the capabilities of popular models such as Flux, Stable Diffusion, and DALL-E, and improves the functionality of ControlNet for fine-grained image editing tasks. To our knowledge, Context Canvas represents the first application of graph-based RAG in enhancing T2I models, representing a significant advancement for producing high-fidelity, context-aware multi-faceted images.
@misc{venkatesh2024contextcanvasenhancingtexttoimage, title={Context Canvas: Enhancing Text-to-Image Diffusion Models with Knowledge Graph-Based RAG}, author={Kavana Venkatesh and Yusuf Dalva and Ismini Lourentzou and Pinar Yanardag}, year={2024}, eprint={2412.09614}, archivePrefix={arXiv}, primaryClass={cs.CV}, url={https://arxiv.org/abs/2412.09614} }
FluxSpace: Disentangled Image Editing in Rectified Flow Models
Kavana Venkatesh, Yusuf Dalva , Pinar Yanardag
In arXiv preprint arXiv:2412.09611
Rectified flow models have emerged as a dominant approach in image generation, showcasing impressive capabilities in high-quality image synthesis. However, despite their effectiveness in visual generation, rectified flow models often struggle with disentangled editing of images. This limitation prevents the ability to perform precise, attribute-specific modifications without affecting unrelated aspects of the image. In this paper, we introduce FluxSpace, a domain-agnostic image editing method leveraging a representation space with the ability to control the semantics of images generated by rectified flow transformers, such as Flux. By leveraging the representations learned by the transformer blocks within the rectified flow models, we propose a set of semantically interpretable representations that enable a wide range of image editing tasks, from fine-grained image editing to artistic creation. This work offers a scalable and effective image editing approach, along with its disentanglement capabilities.
@misc{dalva2024fluxspace, title={FluxSpace: Disentangled Semantic Editing in Rectified Flow Transformers}, author={Yusuf Dalva and Kavana Venkatesh and Pinar Yanardag}, year={2024}, eprint={2412.09611}, archivePrefix={arXiv}, primaryClass={cs.CV} }
2018
Fault Analysis and Predictive Maintenance of Induction Motor using Machine Learning
Kavana Venkatesh, Neethi M
In Proceedings of the International Conference on Electrical, Electronics, Communication, Computer, and Optimization Techniques: ICEECCOT 2018
Induction motors are one of the most crucial electrical equipment and are extensively used in industries in a wide range of applications. This paper presents a machine learning model for the fault detection and classification of induction motor faults by using three phase voltages and currents as inputs. The aim of this work is to protect vital electrical components and to prevent abnormal event progression through early detection and diagnosis. This work presents a fast forward artificial neural network model to detect some of the commonly occurring electrical faults like overvoltage, under voltage, single phasing, unbalanced voltage, overload, ground fault. A separate model free monitoring system wherein the motor itself acts like a sensor is presented and the only monitored signals are the input given to the motor. Limits for current and voltage values are set for the faulty and healthy conditions, which is done by a classifier. Real time data from a 0.33 HP induction motor is used to train and test the neural network. The model so developed analyses the voltage and current values given at a particular instant and classifies the data into no fault or the specific fault. The model is then interfaced with a real motor to accurately detect and classify the faults so that further necessary action can be taken.
@inproceedings{Kavana_2018, title={Fault Analysis and Predictive Maintenance of Induction Motor Using Machine Learning}, url={http://dx.doi.org/10.1109/ICEECCOT43722.2018.9001543}, DOI={10.1109/iceeccot43722.2018.9001543}, booktitle={2018 International Conference on Electrical, Electronics, Communication, Computer, and Optimization Techniques (ICEECCOT)}, publisher={IEEE}, author={Kavana, V and Neethi, M}, year={2018}, month=dec, pages={963–966} }